The Transforming Collections practice researcher in residence programme invited four artists to develop artworks to explore ‘how museums collect and care for collections, how people, artworks and objects gain or lose recognition, and the potential role of new technologies in unearthing these stories’.1
The resulting artworks were exhibited at Tate Modern in October 2024 as part of the Museum x Machine x Me public programme.2
Tan’s installation, Ancestral (r)Evocations 2024 (fig.1), gathers collection data relating to Southeast Asia from the Tate and Wellcome collections. This is fed through a ‘Diagnostic Instrument’, triggering sounds made using fragmented instruments from the region, which reverberate through the physical buildings of these institutions. There are two video components: one features a series of around forty animations triggered directly by the collection data, and the other shows footage of the Diagnostic Instrument being brought into Tate Britain and the Wellcome Collection (fig.2). An additional layer of live, computer-generated sounds, created using machine learning, play back to Tate Modern the sounds of its building. In an artist’s statement, Tan describes her thinking around the project:
Ancestral (r)Evocations is a series of sonic and visual elements brought together within the format of a presentation or event which ‘sounds’ the archive as a method of evocation, diagnosis and making of kin. This is an act of bringing forth or recalling a feeling, memory or image to mind; an invoking of presence to what might nominally be considered invisible, silent, inert and lacking agency. It is the search for kinship, for lost loves, the summoning of those that have been marooned, bereft of companionship, and to pay homage to those that have gone before us. It is also an act of interrogation, a diagnostic probing into the depths of museal collections, processes and their current states of ‘health and well-being’.3
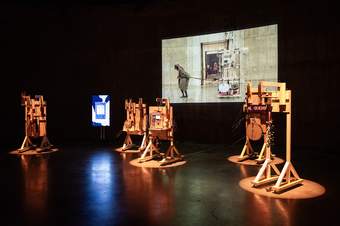
Fig.1
Installation view of Erika Tan, Ancestral (r)Evocations 2024, at Tate Modern, London, 2024
© Erika Tan
Photo © Tate (Ariel Haviland)
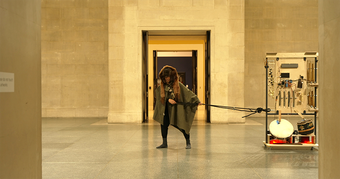
Fig.2
Erika Tan
Ancestral (r)Evocations 2024 (film still)
© Erika Tan
Christopher Griffin: I’d like to start by going back to your initial interest in the Transforming Collections project and the opportunity provided by the residency in particular. What was it about the project – its questions, subject areas or ambitions – that ignited your curiosity and led you to apply?
Erika Tan: I found myself very interested in the project because it related to working with archival material, but also thinking about collections and art histories and national collections. That’s something I’d already been working on. I was also curious about what machine learning and AI would offer to me as an artist who does research within archives and collections. It seemed like a brilliant opportunity to actually move my practice in a different way.
I immediately wanted to look at Southeast Asian material across the project partner collections and bring it all together somehow. That’s a sort of centralising desire – controlling the information, getting information and then mapping, somehow or other – so not decolonial in any shape or form! I was also thinking about the fragmented nature of dispossessed objects, and how often the experience of it – as a researcher, as an artist, as a person of diaspora – is about the gaps and the missing material and the lack of access. Sometimes in projects like this, if partnerships are created, there’s more access; the door has been opened for you. So I think those were my assumptions: that partnerships would mean doors are open, technology would mean doors are open, information’s more readily available. And machine learning might take away some of the laboriousness of this kind of work . It was also interesting and exciting to shift from actual objects and object biographies to thinking about systems and structures that reflect these museums by working with data, numbers and patterns.
One of the things I think I’ve found about this process, though, is there’s very little opportunity for feedback into a system. Because we’re still very external to the museum as a hermetically sealed space. And the machine learning and the AI hasn’t done anything to those spaces for me.
Christopher Griffin: So, on the one hand, the residency allowed you to extend and develop some long-standing interests, especially related to the work of Southeast Asian artists in public and archival collections, while on the other hand it also provided an opportunity to work with machine learning, which represented a brand new approach to research and artmaking for you.
Erika Tan: Yeah, I thought there would be more automation in it. I do a lot of gathering information, and every time I do it, I realise how much I haven’t captured. So it feels quite eclectic. I’ve gone with that, at times. Chance idiosyncratic moments in an archive led to my last piece of work where I was bouncing off different connections. And I was completely registering how much it was my own authoring of those connections, my own speculation. I think when I’m doing it, I understand my position. But I wondered how that might work when my position is less central somehow. I thought that the computer or the technology could somehow eradicate the personal bias, which is what we’ve been talking about – how impossible it is to do that.
Christopher Griffin: Yes, I think all of us on the project have been on a learning curve. We’ve had to reckon with our expectations about what machine learning can do, and what the data might reveal. The idea that machine learning might categorise data in accurate and accessible ways turned out to be a false assumption, for me at least.
Erika Tan: Somehow I thought it could all be brought into play together. So the data from all those different partners would meet seamlessly, and therefore we could step back and just say, ‘Oh wow’. And I wasn’t sure what I’d get. Previously, one of my biggest learnings in terms of museums was that they’re not always in control of the history and the legacy of the collection itself. Provincial museums often hold particularly strange legacies, and their collections demonstrate that. If we shift to national collections, yes, they’re also made through donations and strange acquisitions and stuff like that, but what you might see in there reflects collecting policies.
Christopher Griffin: So how has your understanding of machine learning – its possibilities and limitations – evolved over the course of the residency?
Erika Tan: I think one of the first things I learned on the project was that every time you go to your computer and you want to sign up to something that says, ‘Show us which images are bicycles’, you’re actually participating in a labelling exercise for machine learning. So I think I didn’t realise how much machine learning was going on in my life. I was thinking mostly around ideas of labour and time, around speeding something up and doing something that you couldn’t, as one individual, do on your own.
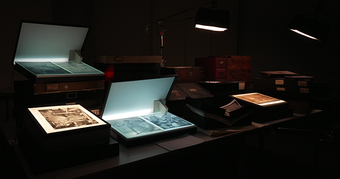
Fig.3
Erika Tan
Ancestral (r)Evocations 2024 (film still)
© Erika Tan
Christopher Griffin: It would be good to know more about the other aspects of the project that interested you, in relation to the kind of research you wanted to conduct, and indeed the kind of art you wanted to make. You mentioned at the beginning that you like to work with collections and archives.
Erika Tan: I think the other thing that seemed to be interesting to me was the different partners that you had on board. The project seemed focused on visual art, fine art and histories of fine art. But within the collections, you also have materials that are ethnographic and material culture. And so I think the two things sitting alongside each other felt really potentially quite rich. I wondered about the relationship between the data on the material cultural sort of side, which represents a more colonial period of collecting, and more contemporary or modern art collecting. In the end, I’m working with Tate and the Wellcome Collection (fig.3). If it had just been, let’s say, Tate only, I think it would have been a very different sort of a project.
I also probably thought that there was an opportunity through the project to be a bit more embedded. I thought, somehow, we might be in the Creative Computing Institute, that there would be much more immediacy to a way of working, so machine learning and AI wouldn’t be so much a subject that we look at, but something we would be actually working with. And that was exciting.
Christopher Griffin: It’s really interesting hearing you talk about your approach to practice-based research. At what point did you start to form ideas for the kind of artwork you wanted to make? Did you have an idea from the beginning, or did you try to keep possibilities open?
Erika Tan: I think it was multi-pronged. That is often how I work. I don’t have a clear idea of what I’m going to do. Very early on, I wanted to make a work that exposed or acknowledged the physicality of the digital space, machine learning and AI, because I think we are often led to believe that it exists as a non-material form. I didn’t really want to make something that could be completely encapsulated and put onto a website, because it determines the kind of engagement somebody has, and because it could then be too easily and nicely wrapped up and contained.
In previous works where I’ve been thinking about archives, often the idea of voice occurs, or the absence of voice and the absence of sound, and who speaks for and how you speak for, and so on. So it seemed like potentially sound would be the focus. Additionally, I have a box of instruments collected by various members of my family from Southeast Asia – some cheap things and others a little bit more hefty, like an Indonesian gamelan. So an idea started to develop, where fragments of instruments might be redeployed or brought together to make something else.
Christopher Griffin: What you were saying about voice and absence chimes directly with one of the project’s central research questions: whose voices are privileged, or cared for, or even heard within collections? Many of us have been addressing this question quite literally in relation to collection interpretation, examining the role that an artist’s own voice plays, or doesn’t play, in artwork narratives. But you also seem to be interested in other kinds of sounds or resonances that invoke artists, beyond simply referring to their words.
Erika Tan: On previous projects, I have come to ‘voice’ in a slightly different way – by looking at and engaging with the actual material. Whereas now I’m trying to avoid the actual material and look at the data. In some ways, if we take the data, we ask: is something present or is it absent? You know, the kind of binary that’s produced by machine learning. At one point, I just wanted to identify which Southeast Asian countries were present in the data and how often. I had hoped to then be able to look across to what material from these countries was present, to see these patterns. That’s not what I’ve done in the end, although that’s where I started.
So the instrument I made for the project is definitely more in line with a metal detector which says, ‘Beep, something’s there’. And it goes ‘bop’ if it’s this country or ‘beep’ if it’s another. When I talk about it now, I think, ‘Well, where’s the machine learning in that?’ But actually, the first line of inquiry, in a way, is, ‘What’s here?’ And it’s taken so much time just to get to ‘What’s here?’
Christopher Griffin: It would be really interesting to know more about how you went about searching for data, and the practical as well as intellectual challenges you faced. What did you discover about public collections data or, perhaps more importantly, what didn’t you discover that you thought you would?
Erika Tan: I was hoping that I could have pulled anything that had a relationship or reference to Southeast Asia. Artworks, object histories, names, titles, dates, etc. I thought that once I got that, I would then explore what to do with it. But data from partner organisations wasn’t forthcoming, so I started to do some research in online catalogues. Then I heard about this idea of scraping websites – going in and getting the stuff that is publicly accessible. There was a realisation that we’re probably not going to get the data in the time to do this project. I also didn’t know how to use machine learning or anything else to help me with the data I did have. I started to work with a computer programmer who could help write programming scripts and scrape data. I realised I had little chance of understanding what this vast amount of data might mean without further programming skills. Again, this was something I hadn’t anticipated.
So while that’s all happening, I then start looking at the material and thinking, ‘So what do I make of this?’ And then I was reminded that the information you get from museums is so arbitrary. It’s arbitrary because, in the process of acquiring the object or the object’s biography, things may or may not get collected and documented. The difference between what you see on the public catalogue and what the curator has on their database is also wildly different. And so you end up recognising that the data isn’t everything. It’s only a tiny portion of it. And that’s frustrating.
Relatedly, there’s a sound component of the work that incorporates some machine learning. We used the prototyping from the project to try and ask a question. Formulating the question was difficult, and insisting on a kind of binary in terms of the answer [such as ‘true’ or ‘false’] was very difficult. There are times that I couldn’t say which one. Was it this one or that one? And the number of times you come across that, and then you realise the nuance is so important, and the computer is not going to recognise the nuance. And so do we actually want the results of the computer? So there is also maybe a disinterest in letting my project be purely computational. But at the beginning, I had the idea that it could be some sort of computer purist sort of thing.
Christopher Griffin: Everything you’ve just described – the frustrations that you’ve shared – exemplifies the critical importance of re-centring the human within machine learning, which is something that we strongly advocate for on this project. We all understand that machine learning can allow us to examine information at scale, but it also raises problems that rely on human computation to address.
Erika Tan: I don’t know when I learned this, but especially for things like architecture, if you get that tiny, tiny bit of a millimetre wrong, and then you extend and you extrapolate from that, that little millimetre becomes a metre’s worth of a problem. I think that’s what I feel has such a potential to happen here. Because even the other day I was looking at the machine’s labelling, and it sort of seemed to suggest that something was problematic. And I was reading it and thinking, ‘Mm-hmm. That doesn’t pan out now.’ I don’t feel myself satisfied by machine learning as a process. But what it did do is, it carved up my data differently. And that carving the data differently allowed it to produce a differentiation in terms of sound that’s produced in the work I’ve made.
Christopher Griffin: It sounds like the process of engaging with machine learning has been quite frustrating, but at the same time productive. That you’ve maybe landed on its artistic value, beyond any scientific potential it may have. Or are you equally frustrated with it artistically?
Erika Tan: I think, for me, I have been frustrated in the past with the discourse around technology that seems to privilege the technical, without acknowledging the human-computer relationship, or human decisions that inform the way in which the technology or indeed the work is actually made. So, for instance, it is frustrating when a project might speak about computer-generated sounds but fails to mention the source material or selection process, or how this piece of computer-generated sound is edited by an artist into a sound work. I have tried to think about how and where my intervention into the computational takes place. The Diagnostic Instrument attempts, in some ways, to be quite straightforward; it reads the collection data and connects this to specific instruments, which are then triggered based on the presence, or not, of a particular quality. Where it gets more complicated, and invested with subjective meaning and personal narrative, is when choices are made about which instruments, or fragments of instruments, to use. The Diagnostic Instrument becomes somewhat anthropomorphic; it looks very DIY, it’s human size, and has got bits of string, tape and other stuff keeping it together, and it’s being physically pulled by a human through the museum space. It’s more Heath Robinson then twenty-first century tech.
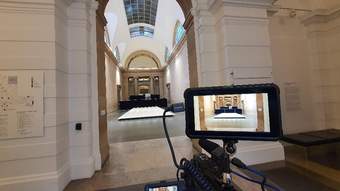
Fig.4
Filming of Vong Phaophanit’s Neon Rice Field 1993 (made with Claire Oboussier) at Tate Britain, for Erika Tan, Ancestral (r)Evocations 2024
© Erika Tan
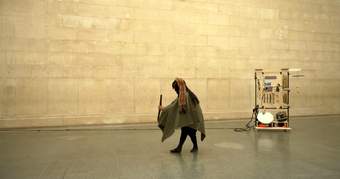
Fig.5
Noel Ed De Leon performing with the Diagnostic Instrument at Tate Britain for Erika Tan, Ancestral (r)Evocations 2024
The other aspect of the work has less to do with technological or computational intelligence than it does with institutional structures and human agency and connections. When I became aware that Vong Phaophanit’s work [Neon Rice Field 1993, made with Claire Oboussier, Tate T14130] was about to be deinstalled at Tate Britain (fig.4), it became an ideal moment to also think about visibility and presence – not just in the data, but in the physical spaces of these institutions and within our own psyches. I took the Diagnostic Instrument to Tate Britain, gathered together Vong’s daughter, Chanti, who is a filmmaker, to document the deinstall of her father’s work, and asked the artist and performer Noel Ed De Leon to help pull the instrument through the galleries to pay homage to another artist, David Medalla. Specific reference was made to David’s work, Sand Machine Bahag – Hari Trance #1 1963–2015, but also to Noel’s own practice, which involves making connections to other artists, including those from the Philippines (fig.5).4 A visitation was also made to the Sphinx 1959 by Singaporean artist Kim Lim, who had featured in my last project Barang-Barang.5 So my interest in what is visible and on display, what is in the stores, and what might have had its opportunity to be seen but will now return to being unseen, is also pitted against what doesn’t want to be seen within the context of the museum. Questions are raised around ownership and repatriation, and then in a counter-direction, around the impulse to be seen and to be represented within these spaces.
So going back to what I said about wanting to control information and centralise it, you can think of it as a kind of neo-colonial enterprise, but you can also think of it the other way round: when you’re fragmented and looking for other fragments to piece things together. So, for me, the system between interpersonal relationships and art generations, the system on the actual instrument itself, and then the potential of the systems of information across these different institutions – they all seem to be doing something that has a kind of resonance with each other.
I did also start to think about an artwork being generative of connections as well. If you understand machine learning as an ability to predict or to generate something, then the artwork also generates these potentials. But, ultimately, it was really difficult to actually do a lot of that machine learning. It felt that, at certain points, the project became more about looking at this process rather than utilising it.
Christopher Griffin: I think many of us on the project have gone on a very similar if not identical journey, from thinking of machine learning as a tool, and being quite suspicious of it, but also hopeful for what it can offer, to then actually grappling with the ethical implications of its practical use, which in turn raises questions, as you have posed yourself, about the relationship between human experience and the computational data that supposedly derives from those experiences.
It would be good to talk now about the final artwork and the presentation you’re planning. How do you envisage all the research and thinking you’ve talked about being manifested? How will the work be experienced? You mentioned at the start that creating something physical was really important to you, but you have also previously expressed anxieties about the longevity of the work.
Erika Tan: At the moment, I’m still thinking that it would be a video work alongside the Diagnostic Instrument, or a series of instruments. So there would be still a live reading of the data by a Raspberry Pi [a small computer consisting of one electronics board] and the sonification of the data from the Tate and Wellcome collections via the instruments. I’m also thinking through the order of the data, which at the moment is randomised so that it will be a continuously changing thing. I’m also looking into other forms of ‘feedback’ that could be included too.
The reason for filming the Diagnostic Instrument in the museum was to activate a feedback loop, or to imply that it functions that way. The film is there to imply that you might have something that goes into a space and disrupts that space, while also somehow looking for and resonating with it, like the human protagonists. I think there might be a possibility that the film could just be there on its own, but at this moment I think the instrument’s actually more important for this project.
In terms of longevity, the question of machine learning and AI is just going to change and rapidly transform, so a piece of work that’s only saying ‘I’m machine learning’ is going to get old very rapidly, I think.
Christopher Griffin: I think the work, as you’ve described it, embodies many of the contradictions and frustrations that we’ve discussed, concerning both machine learning and museum collections, relating to the futile impulse to refine, categorise and control, but in ways that render those contradictions meaningful on a deeply human level.
Erika Tan: You know what? I think the diagnostic machine is me, right? The falling-to-bits, small, diminutive kind of thing, that’s a bit noisy and a bit badly put together. And that maybe there’s a potency in that. Maybe there’s something else that it does too. It doesn’t fully function, it doesn’t quite identify things correctly. But certainly, in the film, when you see it in the Duveen Galleries at Tate Britain, with those huge columns in that big, austere space, there seems to be a poignancy to it, in all its potential falling-to-bits-ness. And there is also, I think, this thing about evoking the spirits, which for me is a bit new. Not necessarily as spirits, but the intangible and the interpretative. All the things that we think machine learning and AI is doing, which is somehow magical.
And the way that we as individuals interpret things, that whole area of perception and interpretation, I think, is uncontrollable. And in a way, this project has pushed me to make something a little bit like that, because actually, my whole encounter with machine learning and AI is just the faultiness of it all, the impossibility of it all, the fact that you’ve still got to make friends with the right people and smile in the right way and write the right email to get the right information. Or you have to scrape it or you have to do something that feels a bit not-quite-right to do. And then you’re back in your make-do-and-mend kind of scenario.
This interview took place online on 9 April 2024. The transcript has been edited for publication by Erika Tan, Christopher Griffin and Andrew Cummings.